Knowledge hub
Read our blog fueled by years of development experience![[object Object]](https://a.storyblok.com/f/86602/304x170/cf91dafa81/home_page_work_together_1.png/m/304x0)
Explore our latest articles
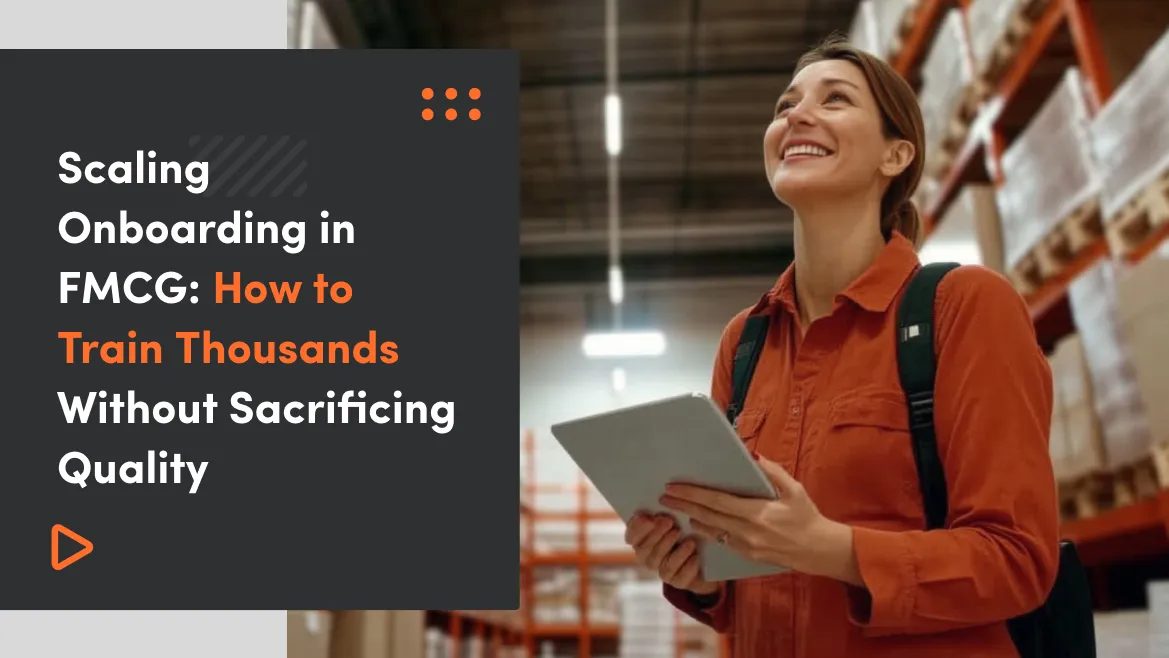
Business
Scaling Onboarding in FMCG: How to Train Thousands Without Sacrificing Quality
Scaling onboarding in FMCG brings a unique tension: the need to move fast without losing depth. As teams grow quickly across multiple regions, it becomes harder to maintain a consistent, high-quality experience for every new hire.
Read more
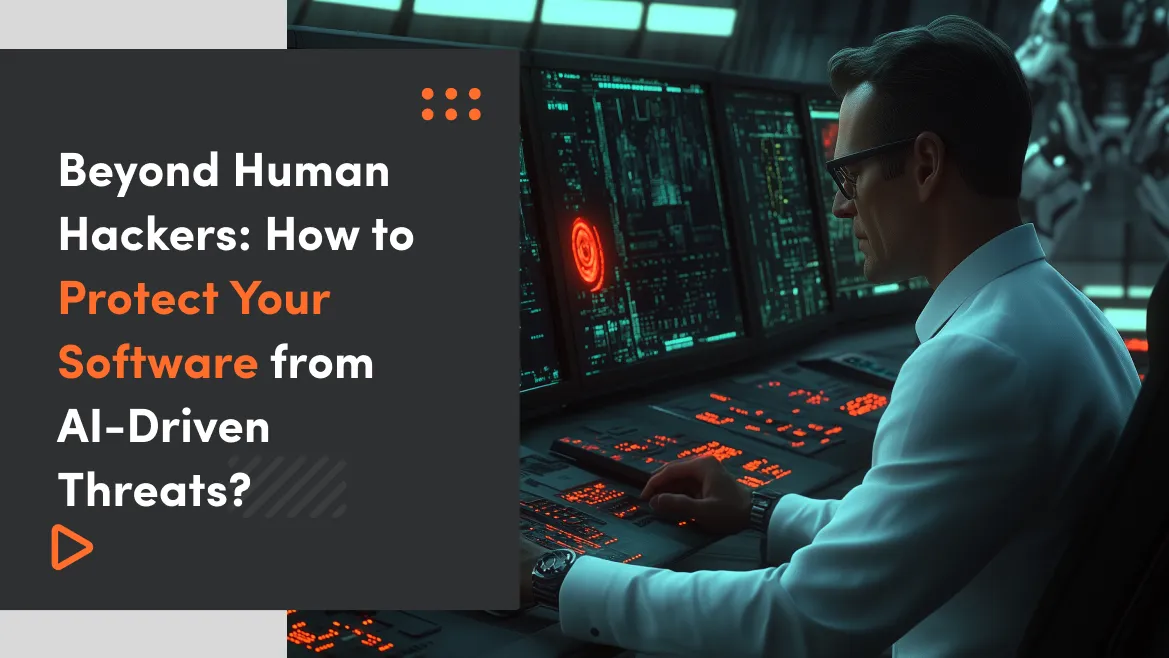
Business
How to Protect Your Software from AI-Driven Threats?
In this article, we’ll explore the rise of AI-driven threats, why traditional defenses fall short, and how to proactively secure your software in this evolving landscape.
Jun 26, 2025
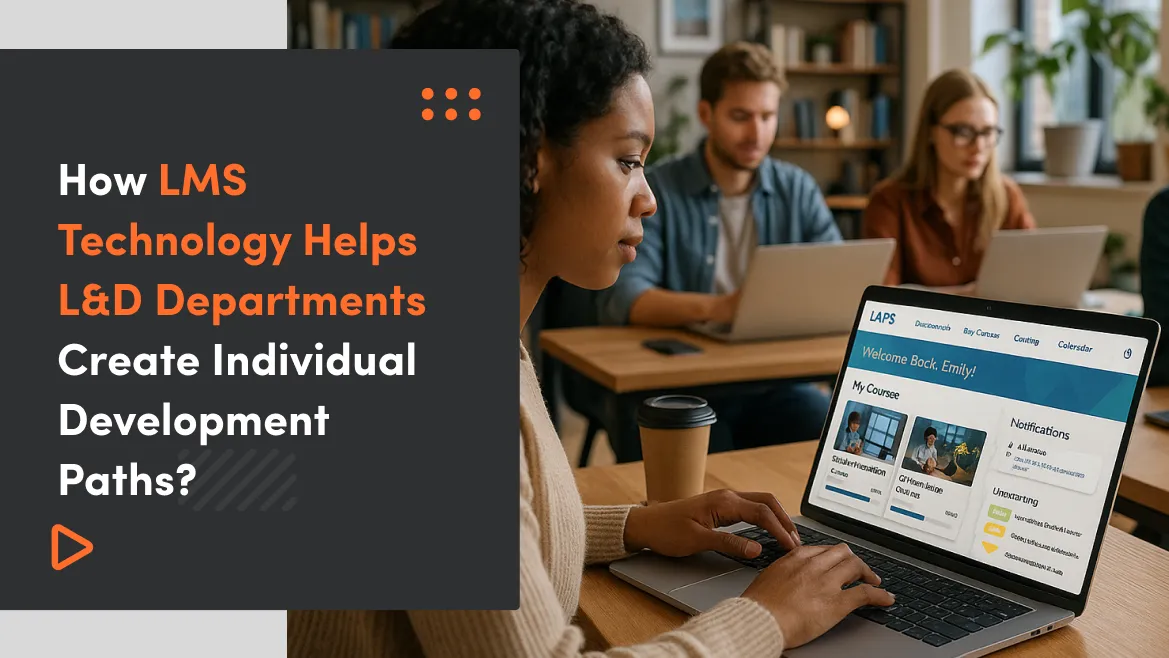
Business
Personalization of Employee Training – How LMS Technology Helps L&D Departments Create Individual Development Paths?
Thankfully, the era of one-size-fits-all training is fading away, replaced by personalized employee training driven by Learning Management System (LMS) technology.
Jun 20, 2025

VIdeo
Is Shape Up Methodology the Answer to Agile and Waterfall Woes?
Ever feel like Agile is making you run in circles with endless meetings and tasks, or that Waterfall is slowing you down with its strict plans and lack of flexibility? What if there's a new way that combines the best of both worlds? It's called Shape Up
Jun 16, 2025

Business
How to Implement an LMS Platform in the L&D Department – Key Aspects of Digital Transformation in Corporate Education
Imagine a scenario where employees effortlessly access engaging training content, HR managers track training effectiveness in real-time, and executives see tangible results from their learning investments.
Jun 11, 2025
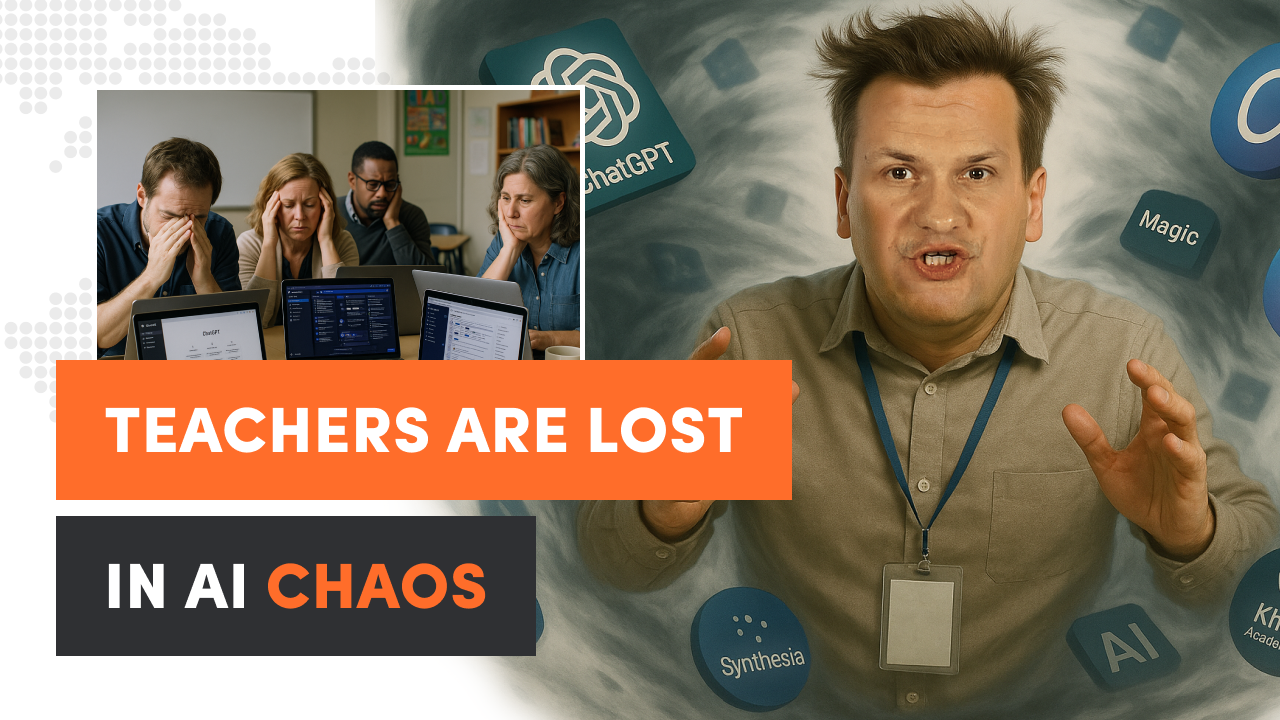
Video
Top Skills Employers Look For in 2025 - What It Means for Education?
Should schools have more control over social media? How do teachers want to teach AI? What do employers really want today? And what does that mean for education?
Jun 6, 2025

Business
Managing Remote Development Teams in 2025 I A Guide
Borders? Time zones? Those are yesterday’s limitations. Welcome to the reality of work in 2025—a world where remote collaboration is no longer a perk but the very heartbeat of your team.Are you ready to master the art of remote leadership and redefine what’s possible for your team?
Jun 3, 2025
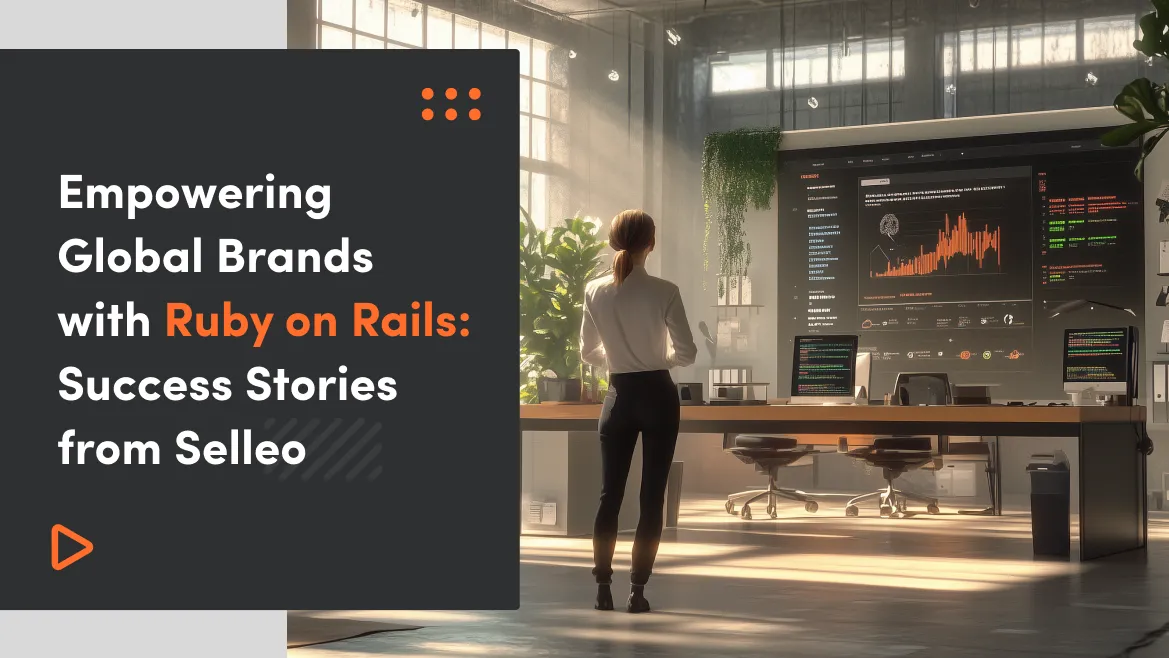
Ruby on Rails
Empowering Global Brands with Ruby on Rails: Success Stories from Selleo
Ruby on Rails (RoR) isn't just a technology; it's the launchpad for innovation. At Selleo, we've spent over fifteen years mastering this powerful web framework.
May 29, 2025

Video
Is Agile dead? Or has AI changed it into something entirely new?
May 21, 2025

Business
How to Foster a Knowledge-Sharing Culture Using Internal Documentation Tools?
How much more could your team achieve if knowledge flowed freely instead of getting trapped in silos? The secret lies in fostering a powerful knowledge-sharing culture supported by effective internal documentation tools.
May 20, 2025
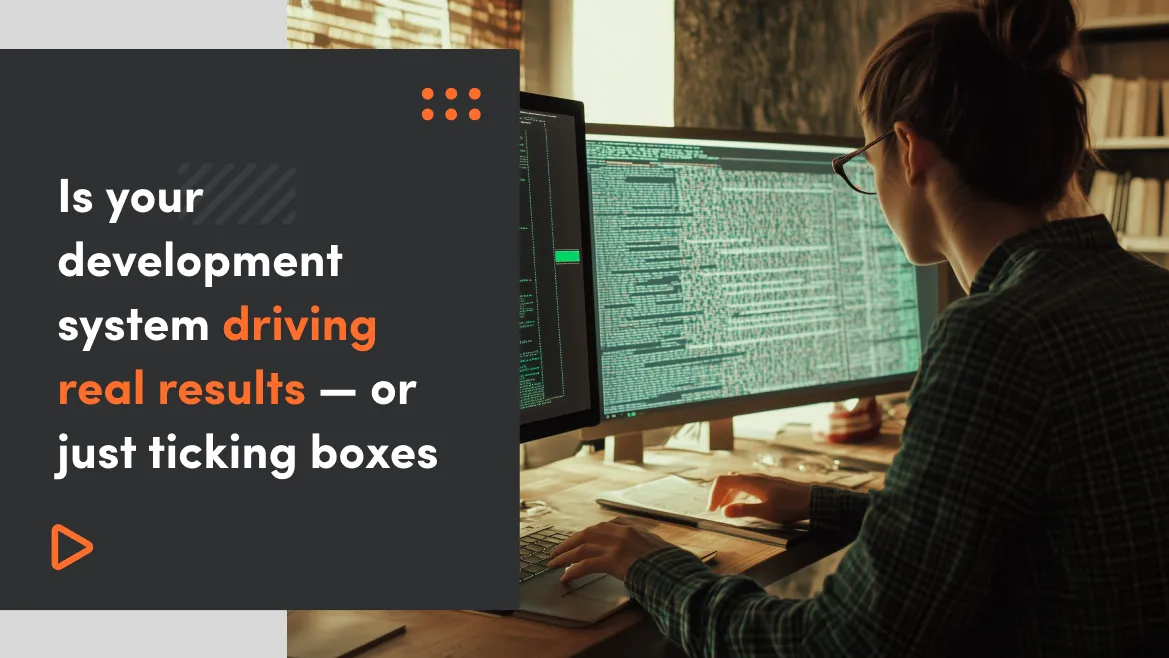
Ruby on Rails
Rewriting a Ruby on Rails App from MongoDB to PostgreSQL: Lessons from a Live Code and Cloud Migration
When our client—a fast-scaling HR and EdTech platform—approached us to rethink their infrastructure, their Ruby on Rails app was already carrying a significant load.
May 15, 2025
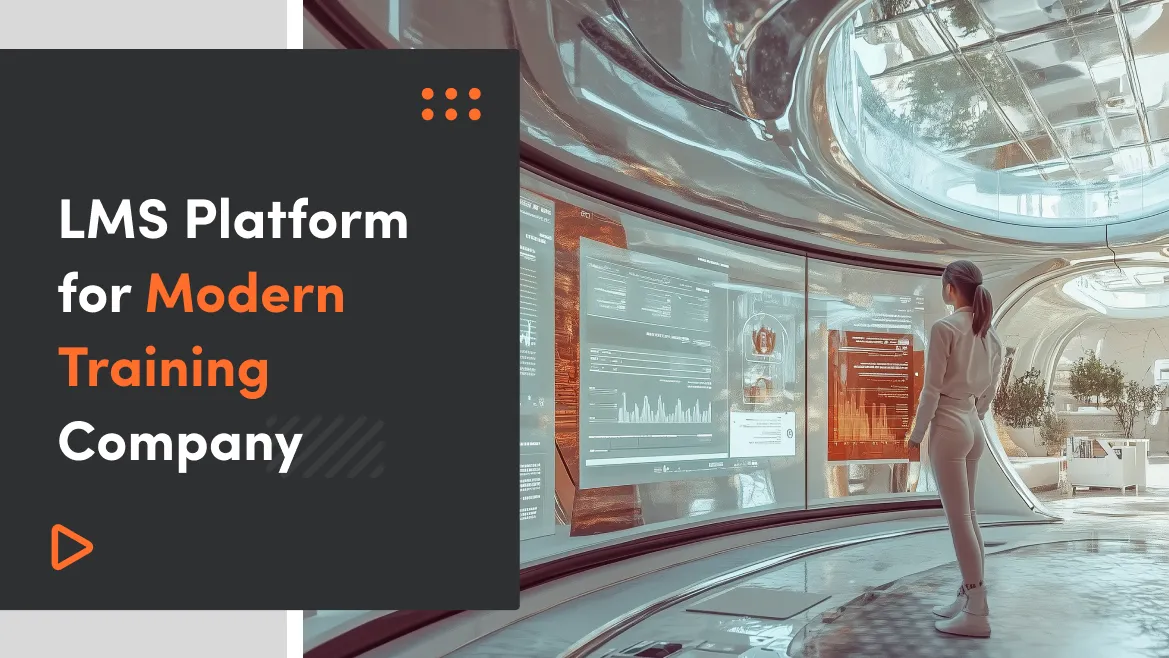
Business
LMS Platform for Modern Training Company – 7 Key Features You Must Know That Will Affect the Monetization of Your Business
Let's dive into the 7 mission-critical features your LMS needs if you’re serious about scaling your training business and maximizing monetization.
May 14, 2025

Video
6 Strategic Pillars of a Successful Digital Transformation in business
Success doesn't happen by accident. It takes clear focus, strategic decisions, and the courage to adapt. In this article, I’ll break down the six core elements that drive digital success
May 9, 2025
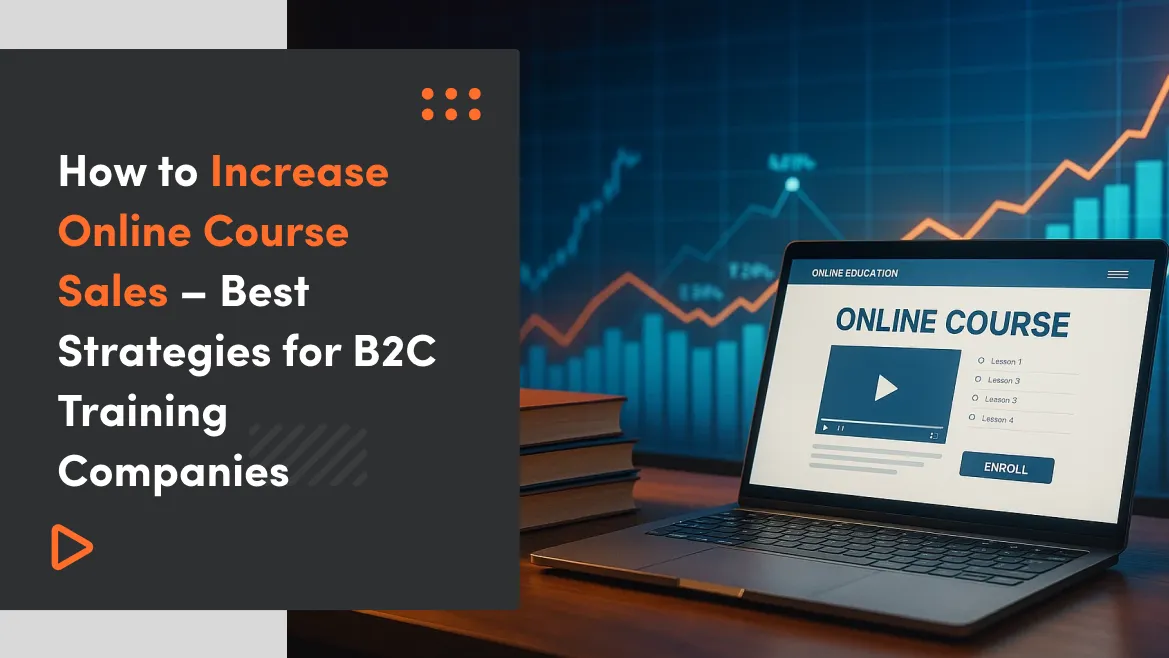
Business
How to Increase Online Course Sales – Best Strategies for B2C Training Companies
With competition growing daily, your course can’t just exist—it needs to stand out, convert, and thrive
May 7, 2025
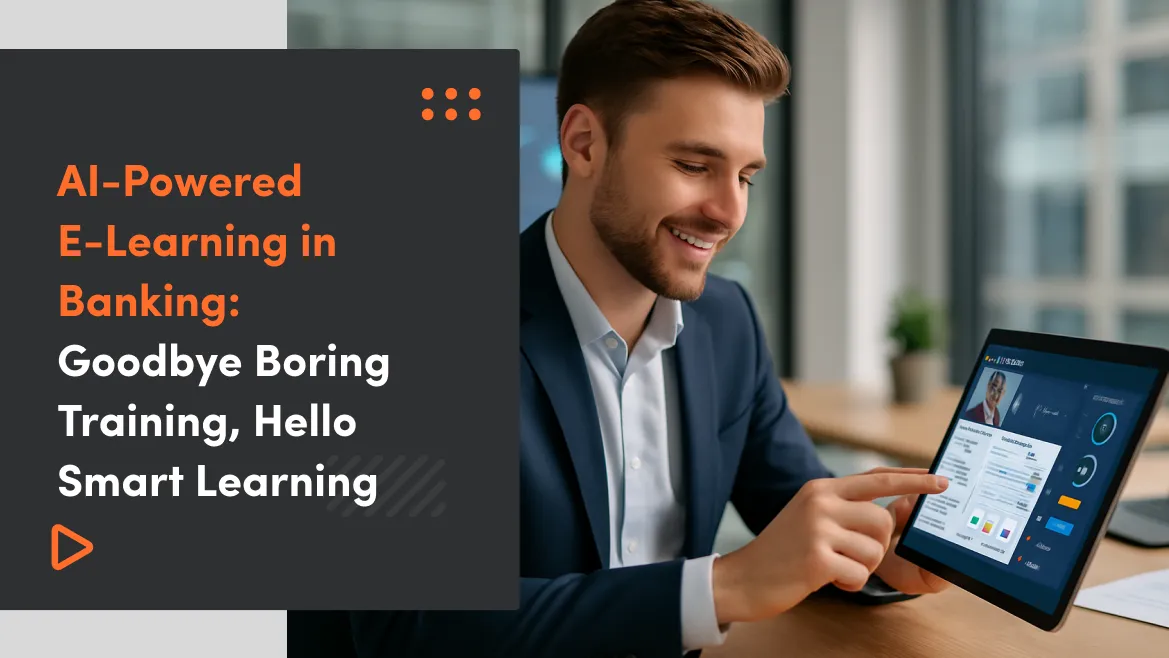
Business
AI-Powered E-Learning in Banking: Goodbye Boring Training, Hello Smart Learning (Featuring Bank of America & HSBC)
Let’s face it: traditional bank training has long been the corporate equivalent of watching paint dry.
Apr 24, 2025
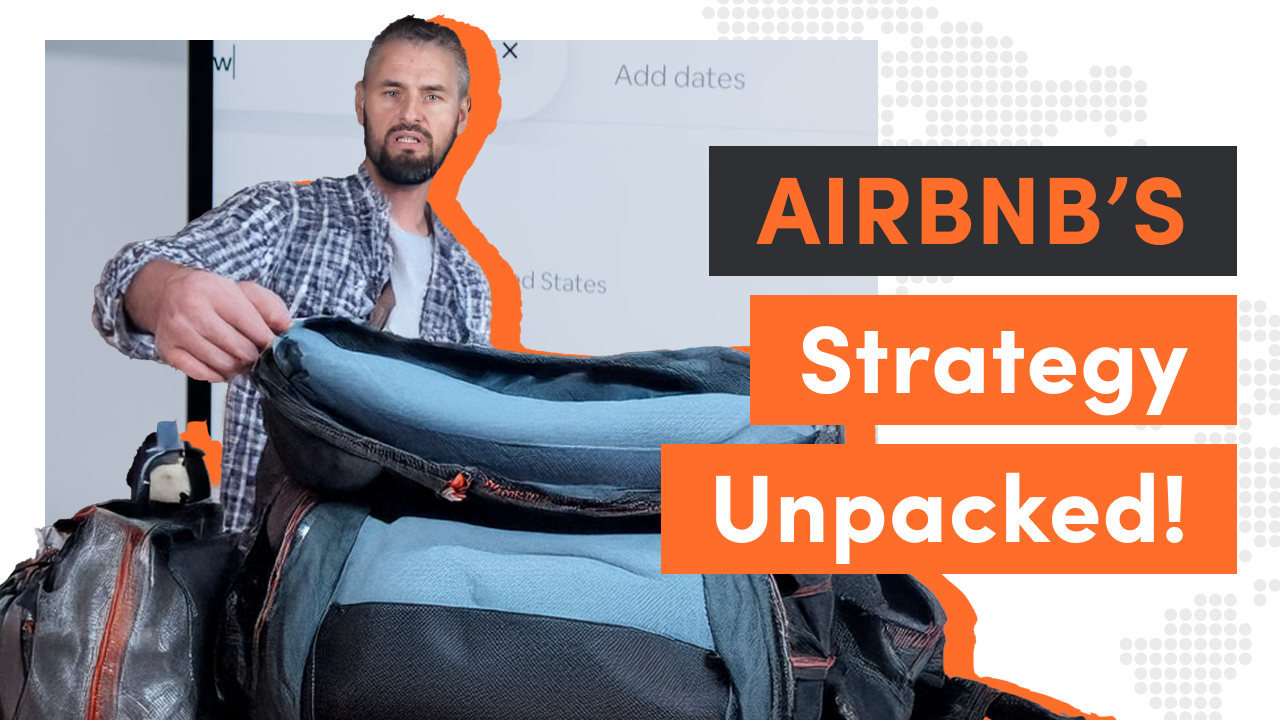
Video
How Two Roommates Built a $86 Billion Company — And What You Can Learn from Airbnb's Strategy
In 2007, two roommates in San Francisco, struggling to make rent, had a simple idea: rent out air mattresses as short-term rentals in their living room to travellers attending a local conference.
Apr 22, 2025
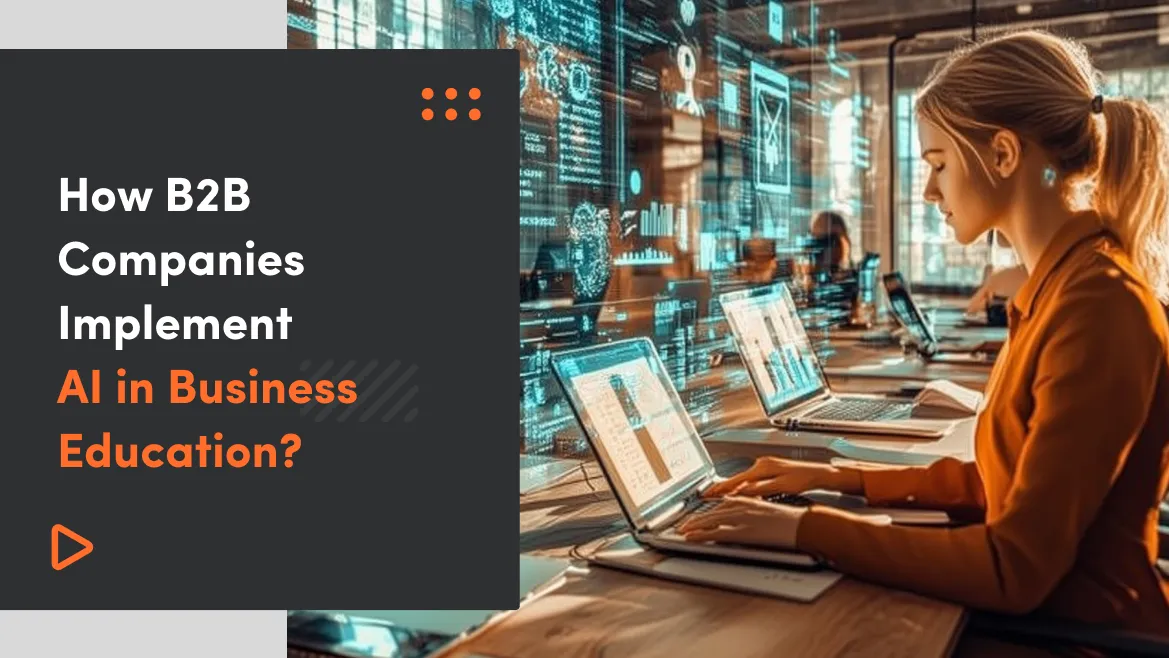
business
How B2B Companies Implement AI in Business Education? Based on True Stories (IBM, Salesforce & Deloitte Case Studies)
Let’s stop thinking about AI in training as “just a tool.” It’s not an upgrade—it’s an evolution. When used right, it becomes the engine of a smarter, faster, more resilient organization.
Apr 17, 2025
SaaS Founder Starter Academy
Everything you need to know to successfully launch your SaaS app.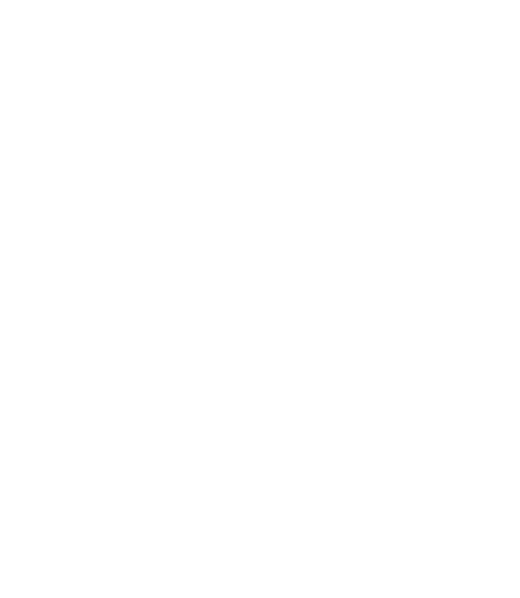



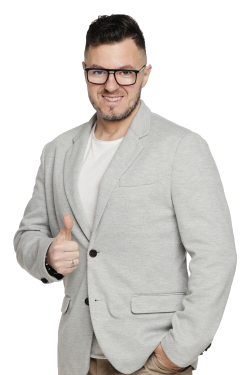
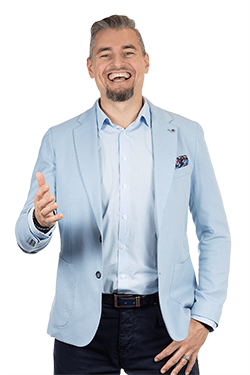

